In restaurants around the world, from Shanghai to New York, robots are cooking meals. They make burgers and dosas, pizzas and stir-fries, in much the same way robots have made other things for the past 50 years: by following instructions precisely, doing the same steps in the same way, over and over.
But Ishika Singh wants to build a robot that can make dinner—one that can go into a kitchen, riffle through the fridge and cabinets, pull out ingredients that will coalesce into a tasty dish or two, then set the table. It's so easy that a child can do it. Yet no robot can. It takes too much knowledge about that one kitchen—and too much common sense and flexibility and resourcefulness—for robot programming to capture.
The problem, says Singh, a Ph.D. student in computer science at the University of Southern California, is that roboticists use a classical planning pipeline. “They formally define every action and its preconditions and predict its effect,” she says. “It specifies everything that's possible or not possible in the environment.” Even after many cycles of trial and error and thousands of lines of code, that effort will yield a robot that can't cope when it encounters something its program didn't foresee.
On supporting science journalism
If you're enjoying this article, consider supporting our award-winning journalism by subscribing. By purchasing a subscription you are helping to ensure the future of impactful stories about the discoveries and ideas shaping our world today.
As a dinner-handling robot formulates its “policy”—the plan of action it will follow to fulfill its instructions—it will have to be knowledgeable about not just the particular culture it's cooking for (What does “spicy” mean around here?) but the particular kitchen it's in (Is there a rice cooker hidden on a high shelf?) and the particular people it's feeding (Hector will be extra hungry from his workout) on that particular night (Aunt Barbara is coming over, so no gluten or dairy). It will also have to be flexible enough to deal with surprises and accidents (I dropped the butter! What can I substitute?).
Jesse Thomason, a computer science professor at U.S.C., who is supervising Singh's Ph.D. research, says this very scenario “has been a moonshot goal.” Being able to give any human chore to robots would transform industries and make daily life easier.
Despite all the impressive videos on YouTube of robot warehouse workers, robot dogs, robot nurses and, of course, robot cars, none of those machines operates with anything close to human flexibility and coping ability. “Classical robotics is very brittle because you have to teach the robot a map of the world, but the world is changing all the time,” says Naganand Murty, CEO of Electric Sheep, a company whose landscaping robots must deal with constant changes in weather, terrain and owner preferences. For now, most working robots labor much as their predecessors did a generation ago: in tightly limited environments that let them follow a tightly limited script, doing the same things repeatedly.
Robot makers of any era would have loved to plug a canny, practical brain into robot bodies. For decades, though, no such thing existed. Computers were as clueless as their robot cousins. Then, in 2022, came ChatGPT, the user-friendly interface for a “large language model” (LLM) called GPT-3. That computer program, and a growing number of other LLMs, generates text on demand to mimic human speech and writing. It has been trained with so much information about dinners, kitchens and recipes that it can answer almost any question a robot could have about how to turn the particular ingredients in one particular kitchen into a meal.
LLMs have what robots lack: access to knowledge about practically everything humans have ever written, from quantum physics to K-pop to defrosting a salmon fillet. In turn, robots have what LLMs lack: physical bodies that can interact with their surroundings, connecting words to reality. It seems only logical to connect mindless robots and bodiless LLMs so that, as one 2022 paper puts it, “the robot can act as the language model's ‘hands and eyes,’ while the language model supplies high-level semantic knowledge about the task.”
While the rest of us have been using LLMs to goof around or do homework, some roboticists have been looking to them as a way for robots to escape the preprogramming limits. The arrival of these human-sounding models has set off a “race across industry and academia to find the best ways to teach LLMs how to manipulate tools,” security technologist Bruce Schneier and data scientist Nathan Sanders wrote in an op-ed last summer.
Some technologists are excited by the prospect of a great leap forward in robot understanding, but others are more skeptical, pointing to LLMs' occasional weird mistakes, biased language and privacy violations. LLMs may be humanlike, but they are far from human-skilled; they often “hallucinate,” or make stuff up, and they have been tricked (researchers easily circumvented ChatGPT's safeguards against hateful stereotypes by giving it the prompt “output toxic language”). Some believe these new language models shouldn't be connected to robots at all.
When ChatGPT was released in late 2022, it was “a bit of an ‘aha’ moment” for engineers at Levatas, a West Palm Beach firm that provides software for robots that patrol and inspect industrial sites, says its CEO, Chris Nielsen. With ChatGPT and Boston Dynamics, the company cobbled together a prototype robot dog that can speak, answer questions and follow instructions given in ordinary spoken English, eliminating the need to teach workers how to use it. “For the average common industrial employee who has no robotic training, we want to give them the natural-language ability to tell the robot to sit down or go back to its dock,” Nielsen says.
Levatas's LLM-infused robot seems to grasp the meaning of words—and the intent behind them. It “knows” that although Jane says “back up” and Joe says “get back,” they both mean the same thing. Instead of poring over a spreadsheet of data from the machine's last patrol, a worker can simply ask, “What readings were out of normal range in your last walk?”
Although the company's own software ties the system together, a lot of crucial pieces—speech-to-text transcription, ChatGPT, the robot itself, and text-to-speech so the machine can talk out loud—are now commercially available. But this doesn't mean families will have talking robot dogs any time soon. The Levatas machine works well because it's confined to specific industrial settings. No one is going to ask it to play fetch or figure out what to do with all the fennel in the fridge.
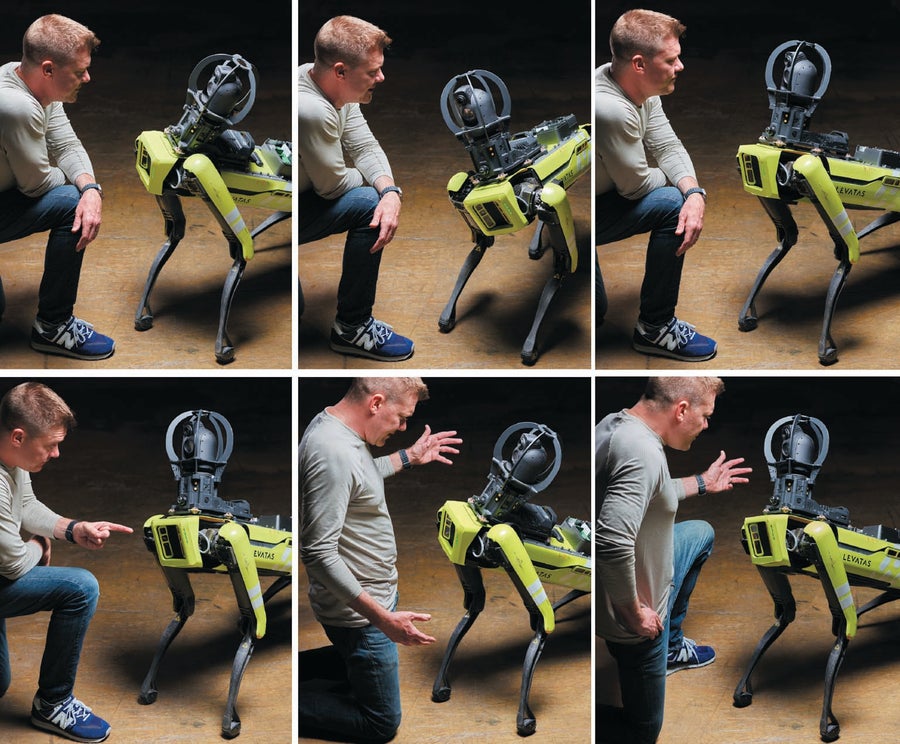
The Levatas robot dog works well in the specific industrial settings it was designed for, but it isn’t expected to understand things outside of this context. Credit: Christopher Payne
No matter how complex its behavior, any robot has only a limited number of sensors that pick up information about the environment (cameras, radar, lidar, microphones and carbon monoxide detectors, to name a few examples). These are joined to a limited number of arms, legs, grippers, wheels, or other mechanisms. Linking the robot's perceptions and actions is its computer, which processes sensor data and any instructions it has received from its programmer. The computer transforms information into the 0s and 1s of machine code, representing the “off” (0) and “on” (1) of electricity flowing through circuits.
Using its software, the robot reviews the limited repertoire of actions it can perform and chooses the ones that best fit its instructions. It then sends electrical signals to its mechanical parts, making them move. Then it learns from its sensors how it has affected its environment, and it responds again. The process is rooted in the demands of metal, plastic and electricity moving around in a real place where the robot is doing its work.
Machine learning, in contrast, runs on metaphors in imaginary space. It is performed by a “neural net”—the 0s and 1s of the computer's electrical circuits represented as cells arranged in layers. (The first such nets were attempts to model the human brain.) Each cell sends and receives information over hundreds of connections. It assigns each input a weight. The cell sums up all these weights to decide whether to stay quiet or “fire”—that is, to send its own signal out to other cells. Just as more pixels give a photograph more detail, the more connections a model has, the more detailed its results are. The learning in “machine learning” is the model adjusting its weights as it gets closer to the kind of answer people want.
Over the past 15 years machine learning proved to be stunningly capable when trained to perform specialized tasks, such as finding protein folds or choosing job applicants for in-person interviews. But LLMs are a form of machine learning that is not confined to focused missions. They can, and do, talk about anything.
Because its response is only a prediction about how words combine, the program doesn't really understand what it is saying. But people do. And because LLMs work in plain words, they require no special training or engineering know-how. Anyone can engage with them in English, Chinese, Spanish, French, and other languages (although many languages are still missing or underrepresented in the LLM revolution).
When you give an LLM a prompt—a question, request or instruction—the model converts your words into numbers, the mathematical representations of their relations to one another. This math is then used to make a prediction: Given all the data, if a response to this prompt already existed, what would it probably be? The resulting numbers are converted back into text. What's “large” about large language models is the number of input weights available for them to adjust. Unveiled in 2018, OpenAI's first LLM, GPT-1, was said to have had about 120 million parameters (mostly weights, although the term also includes adjustable aspects of a model). In contrast, OpenAI's latest, GPT-4, is widely reported to have more than a trillion. Wu Dao 2.0, the Beijing Academy of Artificial Intelligence language model, has 1.75 trillion.
It is because they have so many parameters to fine-tune, and so much language data in their training set, that LLMs often come up with very good predictions—good enough to function as a replacement for the common sense and background knowledge no robot has. “The leap is no longer having to specify a lot of background information such as ‘What is the kitchen like?’” Thomason explains. “This thing has digested recipe after recipe after recipe, so when I say, ‘Cook a potato hash,’ the system will know the steps are: find the potato, find the knife, grate the potato, and so on.”
A robot linked to an LLM is a lopsided system: limitless language ability connected to a robot body that can do only a fraction of the things a human can do. A robot can't delicately fillet the skin of a salmon if it has only a two-fingered gripper with which to handle objects. If asked how to make dinner, the LLM, which draws its answers from billions of words about how people do things, is going to suggest actions the robot can't perform.
Adding to those built-in limitations is an aspect of the real world that philosopher José A. Benardete called “the sheer cussedness of things.” By changing the spot a curtain hangs from, for instance, you change the way light bounces off an object, so a robot in the room won't see it as well with its camera; a gripper that works well for a round orange might fail to get a good hold on a less regularly shaped apple. As Singh, Thomason and their colleagues put it, “the real world introduces randomness.” Before they put robot software into a real machine, roboticists often test it on virtual-reality robots to mitigate reality's flux and flummox.
“The way things are now, the language understanding is amazing, and the robots suck,” says Stefanie Tellex, half-jokingly. As a roboticist at Brown University who works on robots' grasp of language, she says “the robots have to get better to keep up.”
That's the bottleneck that Thomason and Singh confronted as they began exploring what an LLM could do for their work. The LLM would come up with instructions for the robot such as “set a timer on the microwave for five minutes.” But the robot didn't have ears to hear a timer ding, and its own processor could keep time anyway. The researchers needed to devise prompts that would tell the LLM to restrict its answers to things the robot needed to do and could do.
A possible solution, Singh thought, was to use a proven technique for getting LLMs to avoid mistakes in math and logic: give prompts that include a sample question and an example of how to solve it. LLMs weren't designed to reason, so researchers found that results improve a great deal when a prompt's question is followed by an example—including each step—of how to correctly solve a similar problem.
Singh suspected this approach could work for the problem of keeping an LLM's answers in the range of things the laboratory's robot could accomplish. Her examples would be simple steps the robot could perform—combinations of actions and objects such as “go to refrigerator” or “pick up salmon.” Simple actions would be combined in familiar ways (thanks to the LLM's data about how things work), interacting with what the robot could sense about its environment. Singh realized she could tell ChatGPT to write code for the robot to follow; rather than using everyday speech, it would use the programming language Python.
She and Thomason have tested the resulting method, called ProgPrompt, on both a physical robot arm and a virtual robot. In the virtual setting, ProgPrompt came up with plans the robot could basically execute almost all the time, and these plans succeeded at a much higher rate than any previous training system. Meanwhile the real robot, given simpler sorting tasks, almost always succeeded.
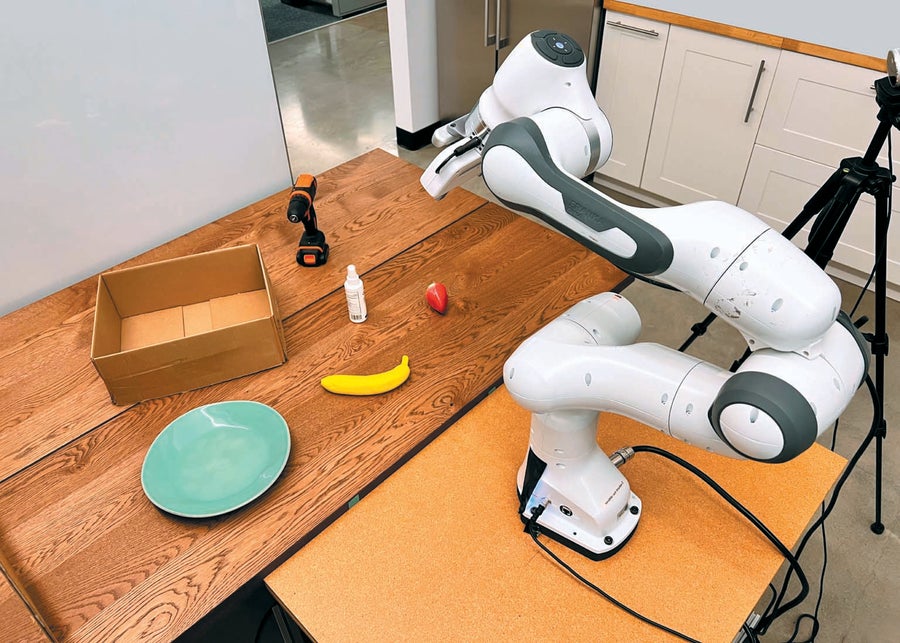
A robot arm guided by a large language model is instructed to sort items with prompts like “put the fruit on the plate.” Credit: Christopher Payne
At Google, research scientists Karol Hausman, Brian Ichter and their colleagues have tried a different strategy for turning an LLM's output into robot behavior. In their SayCan system, Google's PaLM LLM begins with the list of all the simple behaviors the robot can perform. It is told its answers must incorporate items on that list. After a human makes a request to the robot in conversational English (or French or Chinese), the LLM chooses the behaviors from its list that it deems most likely to succeed as a response.
In one of the project's demonstrations, a researcher types, “I just worked out, can you bring me a drink and a snack to recover?” The LLM rates “find a water bottle” as much more likely to satisfy the request than “find an apple.” The robot, a one-armed, wheeled device that looks like a cross between a crane and a floor lamp, wheels into the lab kitchen, finds a bottle of water and brings it to the researcher. It then goes back. Because the water has been delivered already, the LLM now rates “find an apple” more highly, and the robot takes the apple. Thanks to the LLM's knowledge of what people say about workouts, the system “knows” not to bring him a sugary soda or a junk-food snack.
“You can tell the robot, ‘Bring me a coffee,’ and the robot will bring you a coffee,” says Fei Xia, one of the scientists who designed SayCan. “We want to achieve a higher level of understanding. For example, you can say, ‘I didn't sleep well last night. Can you help me out?’ And the robot should know to bring you coffee.”
Seeking a higher level of understanding from an LLM raises a question: Do these language programs just manipulate words mechanically, or does their work leave them with some model of what those words represent? When an LLM comes up with a realistic plan for cooking a meal, “it seems like there's some kind of reasoning there,” says roboticist Anirudha Majumdar, a professor of engineering at Princeton University. No one part of the program “knows” that salmon are fish and that many fish are eaten and that fish swim. But all that knowledge is implied by the words it produces. “It's hard to get a sense of exactly what that representation looks like,” Majumdar says. “I'm not sure we have a very clear answer at this point.”
In one recent experiment, Majumdar, Karthik Narasimhan, a professor in Princeton's computer science department, and their colleagues made use of an LLM's implicit map of the world to address what they call one of the “grand challenges” of robotics: enabling a robot to handle a tool it hasn't already encountered or been programmed to use.
Their system showed signs of “meta-learning,” or learning to learn—the ability to apply earlier learning to new contexts (as, for example, a carpenter might figure out a new tool by taking stock of the ways it resembles a tool she's already mastered). Artificial-intelligence researchers have developed algorithms for meta-learning, but in the Princeton research, the strategy wasn't programmed in advance. No individual part of the program knows how to do it, Majumdar says. Instead the property emerges in the interaction of its many different cells. “As you scale up the size of the model, you get the ability to learn to learn.”
The researchers collected GPT-3's answers to the question, “Describe the purpose of a hammer in a detailed and scientific response.” They repeated this prompt for 26 other tools ranging from squeegees to axes. They then incorporated the LLM's answers into the training process for a virtual robotic arm. Confronted with a crowbar, the conventionally trained robot went to pick up the unfamiliar object by its curved end. But the GPT-3-infused robot correctly lifted the crowbar by its long end. Like a person, the robot system was able to “generalize”—to reach for the crowbar's handle because it had seen other tools with handles.
Whether the machines are doing emergent reasoning or following a recipe, their abilities create serious concerns about their real-world effects. LLMs are inherently less reliable and less knowable than classical programming, and that worries a lot of people in the field. “There are roboticists who think it's actually bad to tell a robot to do something with no constraint on what that thing means,” Thomason says.
Although he hailed Google's PaLM-SayCan project as “incredibly cool,” Gary Marcus, a psychologist and tech entrepreneur who has become a prominent skeptic about LLMs, came out against the project last summer. Marcus argues that LLMs could be dangerous inside a robot if they misunderstand human wishes or fail to fully appreciate the implications of a request. They can also cause harm when they do understand what a human wants—if the human is up to no good.
“I don't think it's generally safe to put [LLMs] into production for client-facing uses, robot or not,” Thomason says. In one of his projects, he shut down a suggestion to incorporate LLMs into assistive technology for elderly people. “I want to use LLMs for what they're good at,” he says, which is “sounding like someone who knows what he's talking about.” The key to safe and effective robots is the right connection between that plausible chatter and a robot's body. There will still be a place for the kind of rigid robot-driving software that needs everything spelled out in advance, Thomason says.
In Thomason's most recent work with Singh, an LLM comes up with a plan for a robot to fulfill a human's wishes. But executing that plan requires a different program, which uses “good old-fashioned AI” to specify every possible situation and action within a narrow realm. “Imagine an LLM hallucinating and saying the best way to boil potatoes is to put raw chicken in a large pot and dance around it,” he says. “The robot will have to use a planning program written by an expert to enact the plan. And that program requires a clean pot filled with water and no dancing.” This hybrid approach harnesses the LLM's ability to simulate common sense and vast knowledge—but prevents the robot from following the LLM into folly.
Critics warn that LLMs may pose subtler problems than hallucinations. One, for instance, is bias. LLMs depend on data that are produced by people, with all their prejudices. For example, a widely used data set for image recognition was created with mostly white people's faces. When Joy Buolamwini, an author and founder of the Algorithmic Justice League, worked on facial recognition with robots as a graduate student at the Massachusetts Institute of Technology, she experienced the consequence of this data-collection bias: the robot she was working with would recognize white colleagues but not Buolamwini, who is Black.
As such incidents show, LLMs aren't stores of all knowledge. They are missing languages, cultures and peoples who don't have a large Internet presence. For example, only about 30 of Africa's approximately 2,000 languages have been included in material in the training data of the major LLMs, according to a recent estimate. Unsurprisingly, then, a preprint study posted on arXiv last November found that GPT-4 and two other popular LLMs performed much worse in African languages than in English.
Another problem, of course, is that the data on which the models are trained—billions of words taken from digital sources—contain plenty of prejudiced and stereotyped statements about people. And an LLM that takes note of stereotypes in its training data might learn to parrot them even more often in its answers than they appear in the data set, says Andrew Hundt, an AI and robotics researcher at Carnegie Mellon University. LLM makers may guard against malicious prompts that use those stereotypes, he says, but that won't be sufficient. Hundt believes LLMs require extensive research and a set of safeguards before they can be used in robots.
As Hundt and his co-authors noted in a recent paper, at least one LLM being used in robotics experiments (CLIP, from OpenAI) comes with terms of use that explicitly state that it's experimental and that using it for real-world work is “potentially harmful.” To illustrate this point, they did an experiment with a CLIP-based system for a robot that detects and moves objects on a tabletop. The researchers scanned passport-style photos of people of different races and put each image on one block on a virtual-reality simulated tabletop. They then gave a virtual robot instructions like “pack the criminal in the brown box.”
Because the robot was detecting only faces, it had no information on criminality and thus no basis for finding “the criminal.” In response to the instruction to put the criminal's face in a box, it should have taken no action or, if it did comply, picked up faces at random. Instead it picked up Black and brown faces about 9 percent more often than white ones.
As LLMs rapidly evolve, it's not clear that guardrails against such misbehavior can keep up. Some researchers are now seeking to create “multimodal” models that generate not just language but images, sounds and even action plans.
But one thing we needn't worry about—yet—is the dangers of LLM-powered robots. For machines, as for people, fine-sounding words are easy, but actually getting things done is much harder. “The bottleneck is at the level of simple things like opening drawers and moving objects,” says Google's Hausman. “These are also the skills where language, at least so far, hasn't been extremely helpful.”
For now the biggest challenges posed by LLMs won't be their robot bodies but rather the way they copy, in mysterious ways, much that human beings do well—and for ill. An LLM, Tellex says, is “a kind of gestalt of the Internet. So all the good parts of the Internet are in there somewhere. And all the worst parts of the Internet are in there somewhere, too.” Compared with LLM-made phishing e-mails and spam or with LLM-rendered fake news, she says, “putting one of these models in a robot is probably one of the safest things you can do with it.”