Deon, a fictional engineer in the 2015 sci-fi film Chappie, wants to create a machine that can think and feel. To this end, he writes an artificial-intelligence program that can learn like a child. Deon's test subject, Chappie, starts off with a relatively blank mental slate. By simply observing and experimenting with his surroundings, he acquires general knowledge, language and complex skills—a task that eludes even the most advanced AI systems we have today.
To be sure, certain machines already exceed human abilities for specific tasks, such as playing games like Jeopardy, chess and the Chinese board game Go. In October 2017 British company DeepMind unveiled AlphaGo Zero, the latest version of its AI system for playing Go. Unlike its predecessor AlphaGo, which had mastered the game by mining vast numbers of human-played games, this version accumulated experience autonomously, by competing against itself. Despite its remarkable achievement, AlphaGo Zero is limited to learning a game with clear rules—and it needed to play millions of times to gain its superhuman skill.
In contrast, from early infancy onward our offspring develop by exploring their surroundings and experimenting with movement and speech. They collect data themselves, adapt to new situations and transfer expertise across domains.
On supporting science journalism
If you're enjoying this article, consider supporting our award-winning journalism by subscribing. By purchasing a subscription you are helping to ensure the future of impactful stories about the discoveries and ideas shaping our world today.
Since the beginning of the 21st century, roboticists, neuroscientists and psychologists have been exploring ways to build machines that mimic such spontaneous development. Their collaborations have resulted in androids that can move objects, acquire basic vocabulary and numerical abilities, and even show signs of social behavior. At the same time, these AI systems are helping psychologists understand how infants learn.
Prediction machine
Our brains are constantly trying to predict the future—and updating their expectations to match reality. Say you encounter your neighbor's cat for the first time. Knowing your own gregarious puppy, you expect that the cat will also enjoy your caresses. When you reach over to pet the creature, however, it scratches you. You update your theory about cuddly-looking animals—surmising, perhaps, that the kitty will be friendlier if you bring it a treat. With goodies in hand, the cat indeed lets you stroke it without inflicting wounds. Next time you encounter a furry feline, you offer a tuna tidbit before trying to touch it.
In this manner, the higher processing centers in the brain continually refine their internal models according to the signals received from the sensory organs. Take our visual systems, which are highly complex. The nerve cells in the eye process basic features of an image before transferring this information to higher-level regions that interpret the overall meaning of a scene. Intriguingly, neural connections also run in the other direction: from high-level processing centers, such as areas in the parietal or temporal cortices, to low-level ones such as the primary visual cortex and the lateral geniculate nucleus [see graphic below]. Some neuroscientists believe that these “downward” connections carry the brain's predictions to lower levels, influencing what we see.
Crucially, the downward signals from the higher levels of the brain continually interact with the “upward” signals from the senses, generating a prediction error: the difference between what we expect and what we experience. A signal conveying this discrepancy returns to the higher levels, helping to refine internal models and generating fresh guesses, in an unending loop. “The prediction error signal drives the system toward estimates of what's really out there,” says Rajesh P. N. Rao, a computational neuroscientist at the University of Washington.
While Rao was a doctoral student at the University of Rochester, he and his supervisor, computational neuroscientist Dana H. Ballard, now at the University of Texas at Austin, became the first to test such predictive coding in an artificial neural network. (A class of computer algorithms modeled on the human brain, neural networks incrementally adapt internal parameters to generate the required output from a given input.) In this computational experiment, published in 1999 in Nature Neuroscience, the researchers simulated neuronal connections in the visual cortex—complete with downward connections carrying forecasts and upward connections bringing sensory signals from the outside world. After training the network using pictures of nature, they found that it could learn to recognize key features of an image, such as a zebra's stripes.
Counting with fingers
A fundamental difference between us and many present-day AI systems is that we possess bodies that we can use to move about and act in the world. Babies and toddlers develop by testing the movements of their arms, legs, fingers and toes and examining everything within reach. They autonomously learn how to walk, talk, and recognize objects and people. How youngsters are able to do all this with very little guidance is a key area of investigation for both developmental psychologists and roboticists. Their collaborations are leading to surprising insights—in both fields.
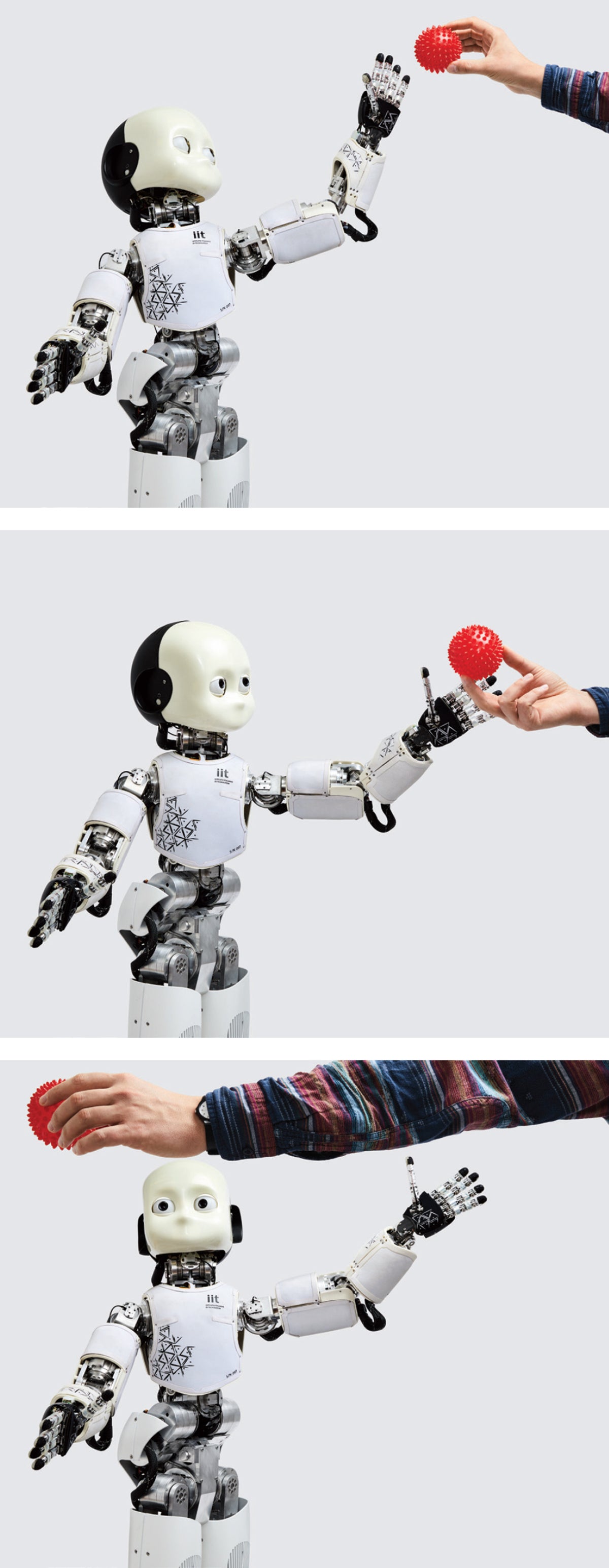
ICUB, an android being studied at the University of Plymouth in England, can learn new words, such as “ball,” more easily if the experimenter consistently places the object at the same location while naming it.
Sun Lee
In a series of pioneering experiments starting in the late 1990s, roboticist Jun Tani, then at Sony Computer Science Laboratories, and others developed a prediction-based neural network for learning basic movements and tested how well these algorithms worked in robots. The machines, they discovered, could attain elementary skills such as navigating simple environments, imitating hand movements, and following basic verbal commands like “point” and “hit.”
More recently, roboticist Angelo Cangelosi of the University of Plymouth in England and Linda B. Smith, a developmental psychologist at Indiana University Bloomington, have demonstrated how crucial the body is for procuring knowledge. “The shape of the [robot's] body, and the kinds of things it can do, influences the experiences it has and what it can learn from,” Smith says. One of the scientists' main test subjects is iCub, a three-foot-tall humanoid robot built by a team at the Italian Institute of Technology for research purposes. It comes with no preprogrammed functions, allowing scientists to implement algorithms specific to their experiments.
In a 2015 study, Cangelosi, Smith and their colleagues endowed an iCub with a neural network that gave it the ability to learn simple associations and found that it acquired new words more easily when objects' names were consistently linked with specific bodily positions. The experimenters repeatedly placed either a ball or a cup to the left or right of the android, so that it would associate the objects with the movements required to look at it, such as tilting its head. Then they paired this action with the items' names. The robot was better able to learn these basic words if the corresponding objects appeared in one specific location rather than in multiple spots.
Interestingly, when the investigators repeated the experiment with 16-month-old toddlers, they found similar results: relating objects to particular postures helped small children learn word associations. Cangelosi's laboratory is developing this technique to teach robots more abstract words such as “this” or “that,” which are not linked to specific things.
Using the body can also help children and robots gain basic numerical skills. Studies show, for instance, that youngsters who have difficulty mentally representing their fingers also tend to have weaker arithmetic abilities. In a 2014 study, Cangelosi and his team discovered that when the robots were taught to count with their fingers, their neural networks represented numbers more accurately than when they were taught using only the numbers' names.
Curiosity engines
Novelty also helps children learn. In a 2015 Science paper, researchers at Johns Hopkins University reported that when infants encounter the unknown, such as a solid object that appears to move through a wall, they explore their violated expectations. In prosaic terms, their in-built drive to reduce prediction errors aids their development.
Pierre-Yves Oudeyer, a roboticist at INRIA, the French national institute for computer science, believes that the learning process is more complex. He holds that kids actively, and with surprising sophistication, seek out those objects in their environment that provide greater opportunities to learn. A toddler, for example, will likely choose to play with a toy car rather than with a 100-piece jigsaw puzzle—arguably because her level of knowledge will allow her to generate more testable hypotheses about the former.
To test this theory, Oudeyer and his colleagues endowed robotic systems with a feature they call intrinsic motivation, in which a decrease in prediction error yields a reward. (For an intelligent machine, a reward can correspond to a numerical quantity that it has been programmed to maximize through its actions.) This mechanism enabled a Sony AIBO robot, a small, puppylike machine with basic sensory and motor abilities, to autonomously seek out tasks with the greatest potential for learning. The robotic puppies were able to acquire basic skills, such as grasping objects and interacting vocally with another robot, without having to be programmed to achieve these specific ends. This outcome, Oudeyer explains, is “a side effect of the robot exploring the world, driven by the motivation to improve its predictions.”
Remarkably, even though the robots went through similar stages of training, chance played a role in what they learned. Some explored a bit less, others a bit more—and they ended up knowing different things. To Oudeyer, these varied outcomes suggest that even with identical programming and a similar educational environment, robots may attain different skill levels—much like what happens in a typical classroom.
More recently, Oudeyer's group used computational simulations to show that robotic vocal tracts equipped with these predictive algorithms (and the proper hardware) could also learn basic elements of language. He is now collaborating with Jacqueline Gottlieb, a cognitive neuroscientist at Columbia University, to investigate whether such prediction-driven intrinsic motivation underlies the neurobiology of human curiosity as well. Probing these models further, he says, could help psychologists understand what happens in the brains of children with developmental disabilities and disorders.
Altruistic androids
The researchers found that even when iCub was not programmed with an intrinsic ability to socialize, the motivation to reduce prediction errors alone led it to behave in a helpful way. For example, after the android was taught to push a toy truck, it might observe an experimenter failing to complete that same action. Often it would move the object to the right place—simply to increase the certainty of the truck being at a given location. Young children might develop in a similar way, believes Nagai, who is currently at the National Institute of Information and Communications Technology in Japan. “The infant doesn't need to have the intention to help other persons,” she argues: the motivation to minimize prediction error can alone initiate elementary social abilities.
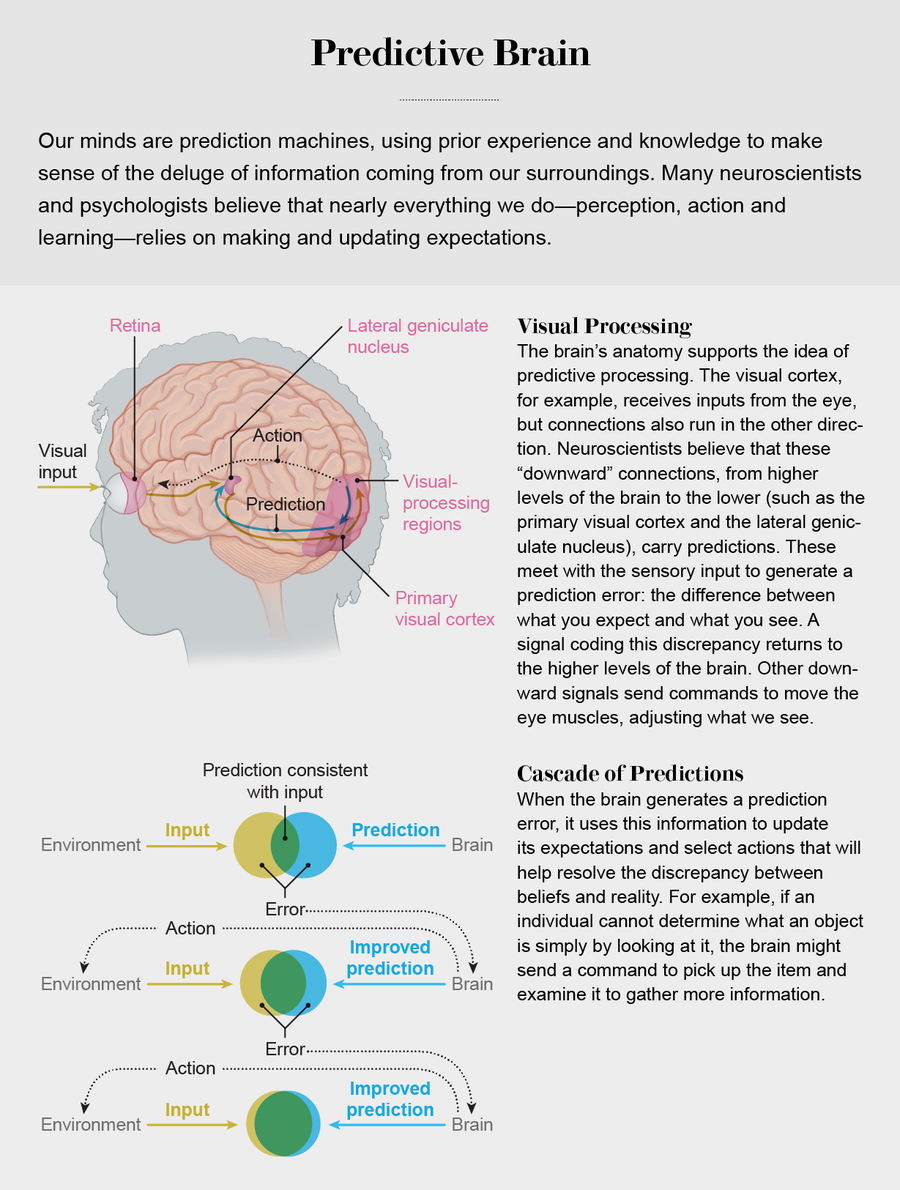
Credit: Mesa Schumacher (brain) and Amanda Montañez (cascade diagram)
Predictive processing may also help scientists understand developmental disorders such as autism. According to Nagai, certain autistic individuals may have a higher sensitivity to prediction errors, making incoming sensory information overwhelming. That could explain their attraction to repetitive behavior, whose outcomes are highly predictable.
Harold Bekkering, a cognitive psychologist at Radboud University in the Netherlands, believes that predictive processing could also help explain the behavior of people with attention deficit hyperactivity disorder. According to this theory, autistic individuals prefer to protect themselves from the unknown, whereas those who have trouble focusing are perpetually attracted to unpredictable stimuli in their surroundings, Bekkering explains. “Some people who are sensitive to the world explore the world, while other people who are too sensitive for the world shield themselves,” he suggests. “In a predictive coding framework, you can very nicely simulate both patterns.” His lab is currently working on using human brain imaging to test this hypothesis.
Nagai hopes to assess this theory by conducting “cognitive mirroring” studies in which robots, equipped with predictive learning algorithms, will interact with people. As the robot and person communicate using body language and facial expressions, the machine will adjust its behaviors to match its partner—thus reflecting the person's preference for predictability. In this way, experimenters can use robots to model human cognition—then examine its neural architecture to try to decipher what is going on inside human heads. “We can externalize our characteristics into robots to better understand ourselves,” Nagai says.
Robots of the future
Studies of robotic children have thus helped answer certain key questions in psychology, including the importance of predictive processing, and of bodies, in cognitive development. “We have learned a huge amount about how complex systems work, how the body matters, [and] about really fundamental things like exploration and prediction,” Smith says.
Robots that can develop humanlike intelligence are far from becoming a reality, however: Chappie still belongs in the realm of science fiction. To begin with, scientists need to overcome technical hurdles, such as the brittle bodies and limited sensory capabilities of most robots. (Advances in areas such as soft robotics and robot vision may help this happen.) Far more challenging is the incredible intricacy of the brain itself. Despite efforts on many fronts to model the mind, scientists are far from engineering a machine to rival it. “I completely disagree with people who say that in 10 or 20 years we'll have machines with human-level intelligence,” Oudeyer says. “I think it's showing a profound misunderstanding of the complexity of human intelligence.”
Moreover, intelligence does not merely require the right machinery and circuitry. A long line of research has shown that caregivers are crucial to children's development. “If you ask me if a robot can become truly humanlike, then I'll ask you if somebody can take care of a robot like a child,” Tani says. “If that's possible, then yes, we might be able to do it, but otherwise, it's impossible to expect a robot to develop like a real human child.”
The process of gradually accumulating knowledge may also be indispensable. “Development is a very complex system of cascades,” Smith says. “What happens on one day lays the groundwork for [the next].” As a result, she argues, it might not be possible to build human-level artificial intelligence without somehow integrating the step-by-step process of learning that occurs throughout life.
Soon before his death, Richard Feynman famously wrote: “What I cannot create, I do not understand.” In Tani's 2016 book, Exploring Robotic Minds, he turns his concept around, saying, “I can understand what I can create.” The best way to understand the human mind, he argues, is to synthesize one.
One day humans may succeed in creating a robot that can explore, adapt and develop just like a child, perhaps complete with surrogate caregivers to provide the affection and guidance needed for healthy growth. In the meantime, childlike robots will continue to provide valuable insights into how children learn—and reveal what might happen when basic mechanisms go awry.